Using machine to cluster and predict the learning pattern of university students
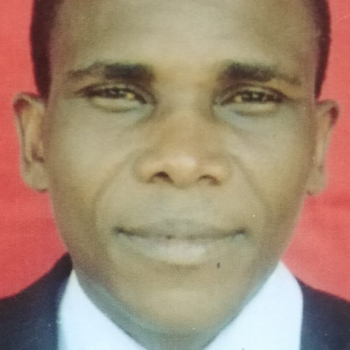
+233 (0) 249565970 | |
gkbada@uew.edu.gh |
Using machine to cluster and predict the learning pattern of university students
The devastating COVID-19 pandemic has brought rapid transformation to the educational sector with the increasing integration of E-learning systems to complement the traditional classroom. Whether the lesson is online or in the conventional classroom, understanding students' learning styles is relevant, especially in active learning and collaborative projects. The study employed the k-modes clustering algorithm on thirty seven relevant categorical attributes that reveal students' hidden learning styles. The purposive sampling approach was used to collect data from level 100 and 200 students in the Department of ICT Education, University of Education, Winneba. The elbow method implementation of cluster identification led to the formation of three distinct clusters. The first cluster consists of visual and social learning. The second cluster members are primarily verbal and solitary learners, whiles the third cluster members are visual, social and logical learners. In addition to clustering, a classification model was built using the 10-fold cross-validation and Pearson’s correlation feature selection evaluator. The ensemble AdaBoost (RF) emerged as the best classifier with an accuracy of 61.77%.